Introduction to Generative AI - Everything you need to know
- Deepak Subramanian
- May 20, 2023
- 3 min read
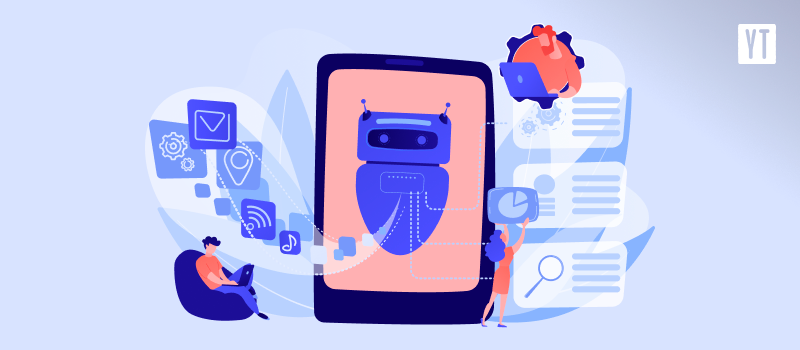
What is Generative AI?
Generative AI enables users to generate new content based on a variety of inputs quickly. Inputs and outputs to these models can include text, images, sounds, animation, 3D models, or other data types.
How Does Generative AI Work?
Generative AI models use neural networks to identify the patterns and structures within existing data to generate new and original content.
One of the breakthroughs with generative AI models is the ability to leverage different learning approaches, including unsupervised or semi-supervised learning, for training. This has allowed organisations to leverage a large amount of unlabeled data more easily and quickly to create foundation models. As the name suggests, foundation models can be used as a base for AI systems performing multiple tasks.
Examples of foundation models include GPT-3 and Stable Diffusion, which allow users to leverage the power of language. For instance, popular applications like ChatGPT, which draws from GPT-3, enable users to generate an essay based on a short text request. On the other hand, Stable Diffusion allows users to create photorealistic images given text input.
What are the Applications of Generative AI?
Generative AI is a powerful tool for streamlining the workflow of creatives, engineers, researchers, scientists, and more. The use cases and possibilities span all industries and individuals.
Generative AI models can take inputs such as text, image, audio, video, and code and generate new content into any modalities mentioned. For example, it can turn text inputs into an image, rotate an image into a song, or turn video into text.
Here are the most popular generative AI applications:
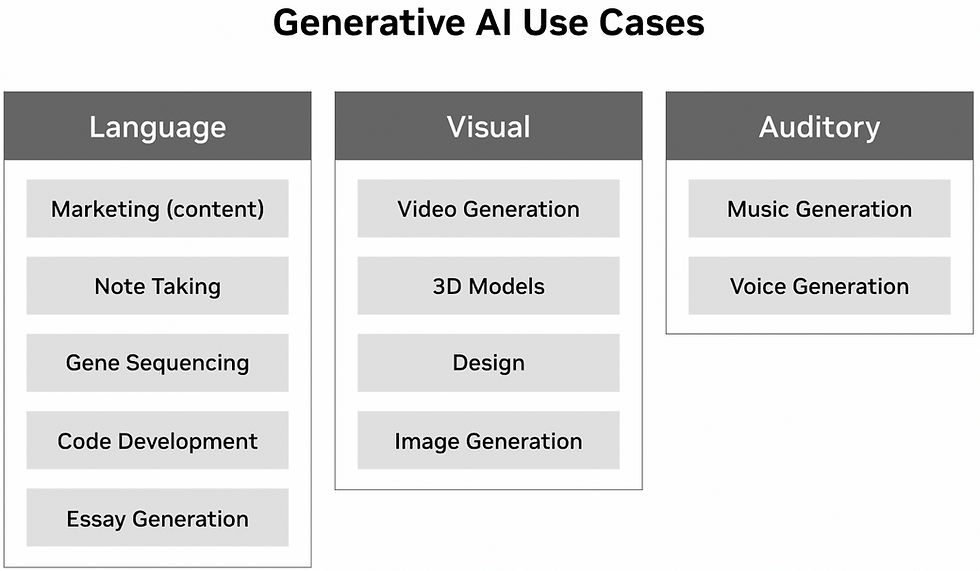
Language: Text is at the root of many generative AI models and is considered the most advanced domain. One of the most famous examples of language-based generative models is called large language models (LLMs). Large language models are being leveraged for various tasks, including essay generation, code development, translation, and understanding of genetic sequences.
Audio: Music, audio, and speech are also emerging fields within generative AI. Examples include models developing songs and snippets of audio clips with text inputs, recognising objects in videos and creating accompanying noises for different video footage, and even making custom music.
Visual: One of the most popular applications of generative AI is within the realm of images. This encompasses the creation of 3D images, avatars, videos, graphs, and other illustrations. There’s flexibility in generating images with different aesthetic styles and techniques for editing and modifying rendered visuals. Generative AI models can create graphs that show new chemical compounds and molecules that aid in drug discovery, create realistic images for virtual or augmented reality, produce 3D models for video games, design logos, enhance or edit existing images, and more.
Synthetic data: Synthetic data is handy for training AI models when data doesn’t exist, is restricted, or cannot address corner cases with the highest accuracy. Developing synthetic data through generative models is perhaps one of the most impactful solutions for overcoming the data challenges of many enterprises. It spans all modalities and use cases and is possible through a process called label-efficient learning. Generative AI models can reduce labeling costs by automatically producing additional augmented training data or by learning an internal representation of the data that facilitates training AI models with less labeled data.
What are the Benefits of Generative AI?
Generative AI is essential for several reasons. Some of the critical benefits of generative AI include:
Generative AI algorithms can be used to create new, original content, such as images, videos, and text, that’s indistinguishable from human-generated content. This can be useful for entertainment, advertising, and creative arts applications.
Generative AI algorithms can improve the efficiency and accuracy of existing AI systems, such as natural language processing and computer vision. For example, generative AI algorithms can be used to create synthetic data that can be used to train and evaluate other AI algorithms.
Generative AI algorithms can explore and analyse complex data in new ways, allowing businesses and researchers to uncover hidden patterns and trends that may not be apparent from the raw data alone.
Generative AI algorithms can help automate and accelerate various tasks and processes, saving time and resources for businesses and organisations.
Overall, generative AI has the potential to impact a wide range of industries and applications significantly and is an essential area of AI research and development.
Comments